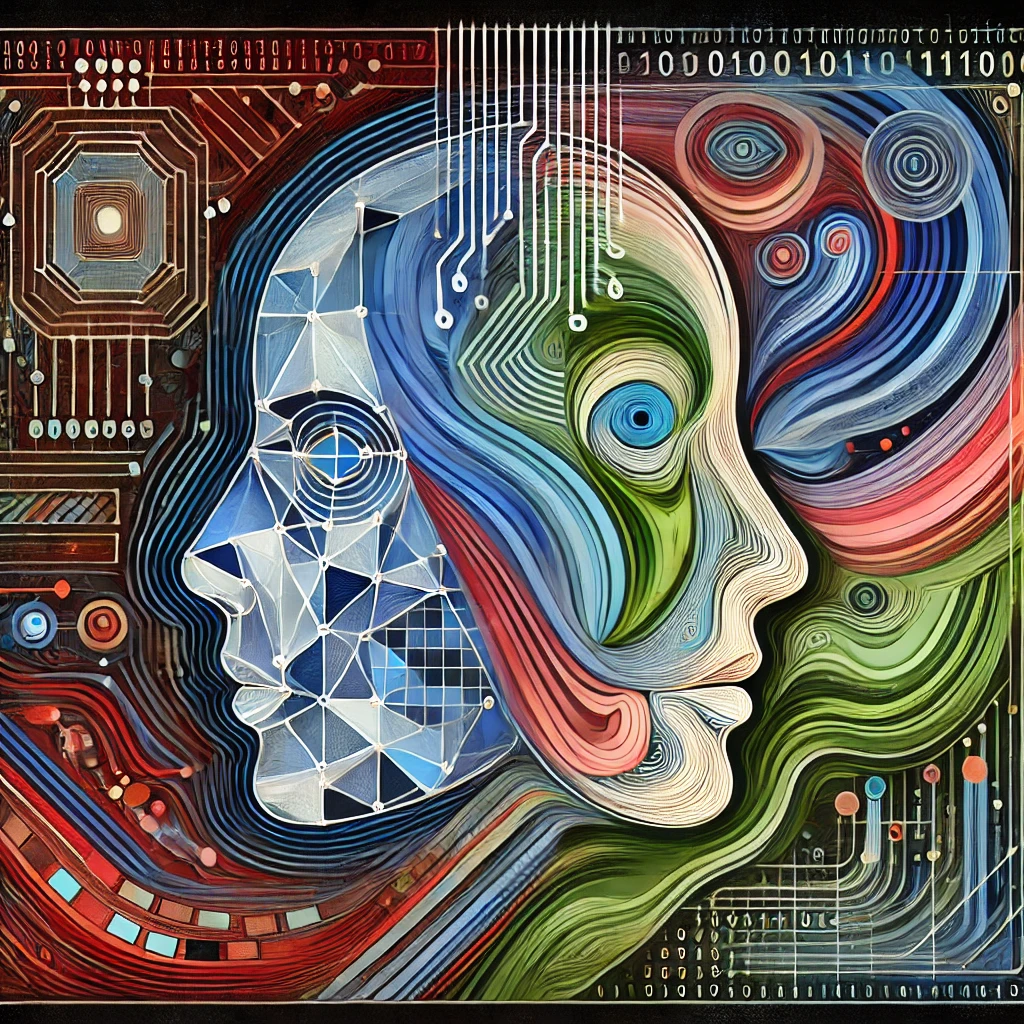
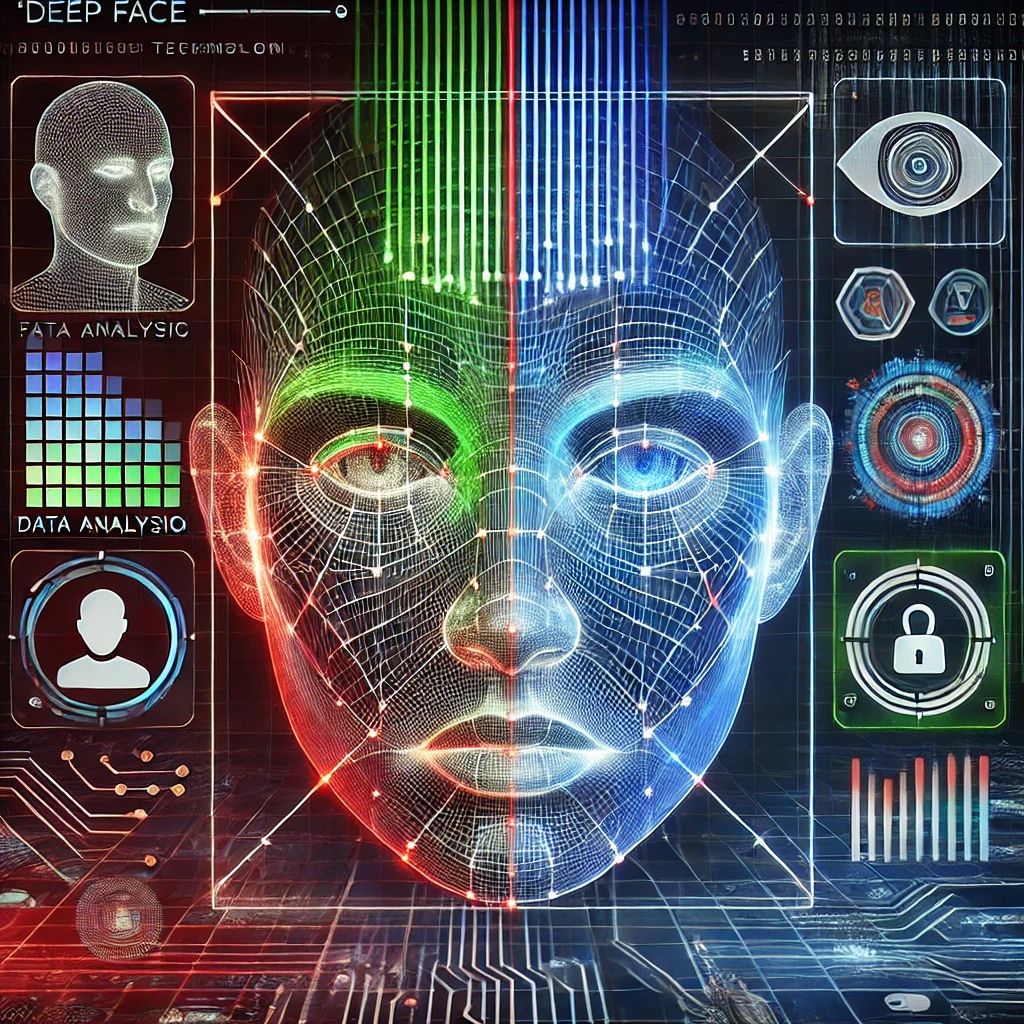
Welcome to our article series where we decode the world’s most important research papers on Generative AI using chatbots. We’ll continue our journey with the influential paper “DeepFace: Closing the Gap to Human-Level Performance in Face Verification,” which showcases a remarkable leap in facial recognition technology. As mentioned in the introductory article (if you missed it, check it out here), the goal here is to make the most significant research papers on AI less mysterious and more approachable to everyone. And the best part is that throughout this article series, we are using AI to explain itself.Read More
ㅤ
WHAT IS THIS STUDY ABOUT?
ㅤ
This study, published in 2014, aimed to improve face recognition technology to be as accurate as humans. The goal was to make face recognition better by using a special type of artificial intelligence called a deep neural network (DNN) along with a 3D modeling technique to align faces accurately. The researchers believed that combining these two methods would significantly boost the accuracy and reliability of face recognition systems. They trained the DNN on a massive collection of four million labeled facial images and used 3D modeling to ensure each face was positioned correctly before analysis. The results were impressive, with the system achieving 97.35% accuracy on a standard test set of face images, which is very close to human performance and a 27% improvement over previous methods. The innovative part of this study was how they combined 3D face alignment with deep learning, enabling the system to recognize faces accurately in various real-world scenarios, making it a major breakthrough in face recognition technology.
ㅤ
Let’s break down each aspect of this paper to understand it better.
ㅤ
First, let’s ask GPT what the DNN is?
ㅤ
PROMPT: Using Layman’s term briefly explain what the DNN is? Picture it using a simple comparison that a 4grader would understnad
ㅤ
GPT response:
“A Deep Neural Network (DNN) is like a super-smart computer brain made up of many connected layers that help it learn to recognize patterns. Imagine it as a giant LEGO tower, where each layer of LEGO bricks helps the tower understand something new. The first layer might recognize simple shapes, like squares and circles. The next layers build on that, recognizing more complex things like faces, by putting together all the simple shapes it learned earlier. Just like how a tower gets taller and stronger with more LEGO bricks, the DNN gets smarter and better at recognizing things with more layers.”
ㅤ
Brilliant, that makes it a lot easier to apprehend.
HOW WAS THE STUDYCONDUCTED?
ㅤ
It was conducted with the hypothesis that integrating 3D face modeling with a deep neural network (DNN) would significantly enhance the accuracy and reliability of face recognition systems. To test this, the researchers employed a two-part method. First, the researchers gathered a massive dataset of four million labeled facial images from various sources, ensuring a diverse and comprehensive training set. Then they made sure to align facial images accurately, ensuring that each face was positioned in a standardized way regardless of variations in angle, lighting, or expression. This “standardized way” refers to a process called 3D face alignment, where the 2D facial images were mapped onto a 3D model. To better understand, imagine you have a bunch of photos of friends taken at different angles and in various lighting conditions. To create a school yearbook, you want all the photos to look similar, with everyone facing forward and evenly lit. So, you use a tool that adjusts each photo, rotating and brightening them so all faces appear straight-on and well-lit, as if everyone took their photo under the same conditions. This alignment makes it easier to recognize and compare faces, just like the 3D face alignment process does for the DNN.
This technique adjusts the images so that key facial features—like the eyes, nose, and mouth—are consistently positioned in the same locations across all images. By doing this, the researchers could create a uniform dataset where all faces appeared as if they were looking straight ahead under similar lighting conditions, which is crucial for training the DNN effectively and improving recognition accuracy.
ㅤ
UNVEILING DEEPFACE: A MILESTONE IN FACE VERIFICATION
ㅤ
In this groundbreaking study by Facebook AI Research, DeepFace has emerged as a pivotal innovation in face recognition, achieving near-human accuracy. This remarkable advancement leverages deep learning and 3D face modeling to push the boundaries of what machines can accomplish in recognizing faces under diverse and unconstrained conditions.
ㅤ
WHAT IS THE CORE of DeepFace?
ㅤ
“An ideal face classifier would recognize faces in accuracy that is only matched by humans,” the researchers assert, and DeepFace strives to meet this ideal. The key to DeepFace’s success lies in its unique combination of a deep neural network (DNN) and a sophisticated 3D face alignment method. The previously explained DNN – complex structure with over 120 million parameters, is meticulously trained on an extensive dataset of four million facial images.
ㅤ
So, let’s ask the question we’are all thinking about.
ㅤ
AI VS HUMAN: IS DeepFace AS ACCURATE AS HUMAN?
ㅤ
DeepFace’s performance on the Labeled Faces in the Wild (LFW) dataset is particularly impressive, achieving a 97.35% accuracy rate. This benchmark dataset is widely recognized in the field for evaluating face verification systems. Imagine LFW as a huge yearbook filled with thousands of photos of celebrities taken in various settings—some are well-lit studio shots, while others are candid photos from parties or outdoor events. The challenge is to accurately identify whether two photos show the same person despite these variations. DeepFace’s ability to reduce the error rate by more than 27% means it can match faces with an accuracy close to that of a human, which was previously thought to be a far-off goal. Studies have shown that human accuracy on the LFW dataset is around 97.53% for cropped face images, which is slightly higher than DeepFace’s performance. This suggests that while DeepFace is incredibly accurate, humans still have a slight edge, especially in controlled conditions.
ㅤ
“Face recognition in unconstrained images is at the forefront of the algorithmic perception revolution,”
ㅤ
the authors highlight. This leap in accuracy is not just a technical achievement; it signifies a profound step forward in how we interact with and utilize artificial intelligence in daily life. In medicine, this technology can be used to quickly and accurately identify patients, ensuring the right treatments are given. In mechanics, it could help in identifying and cataloging parts, quickly. Additionally, this technique can be applied to other objects as well, streamlining inventory management and quality control processes across various industries. Corporate businesses can use advanced face recognition for secure access control and personalized customer service. Passport control and world security would benefit immensely from this, as it could streamline identification processes at borders and enhance security measures by accurately identifying individuals in crowded places. By approaching human-level accuracy, DeepFace is transforming these real-world applications, making interactions more secure, efficient, and reliable.
ㅤ
Let’s stop for a moment and take a second to look at it from a skeptical and more pessimistic perspective.
ㅤ
PROMPT: What are the potential risks associated with this technology advancement, consider real-world situations
ㅤ
GPT response: “DeepFace’s advanced face recognition technology, while groundbreaking, carries several potential risks when viewed from a pessimistic and skeptical perspective. In real-world situations, the misuse of this technology could lead to significant privacy violations. For instance, in corporate environments, employees might be constantly monitored without their consent, leading to a loss of privacy and creating a culture of distrust.
ㅤ
In law enforcement and security, the potential for false positives—where innocent people are incorrectly identified as suspects—could lead to wrongful detentions or invasions of privacy. This is particularly concerning in scenarios like airport security or public surveillance, where large-scale deployment of face recognition could result in frequent and unjustified intrusions into individuals’ lives.
ㅤ
Moreover, the technology could be exploited for mass surveillance by authoritarian regimes, where it might be used to track and suppress dissent, monitor activists, or target minority groups. The deployment of such systems without stringent checks and balances could lead to significant human rights abuses.
ㅤ
Another risk is the potential for data breaches. The vast amounts of personal data required to train and operate face recognition systems could become targets for hackers, leading to identity theft or other forms of cybercrime. Additionally, there is the concern of algorithmic bias; if the training data is not adequately diverse, the system might perform poorly on certain demographic groups, leading to discrimination and reinforcing societal biases.
ㅤ
Finally, the reliance on face recognition technology could lead to complacency, where over-reliance on automated systems diminishes human judgment and oversight. This can result in a failure to question or verify the system’s accuracy, further exacerbating the potential for errors and misuse.
ㅤ
In summary, while DeepFace technology holds significant promise, its advancement comes with substantial risks that need to be carefully managed to avoid privacy infringements, discrimination, misuse, and other ethical concerns.”
.ㅤ
I think it is best summed up using a famous comic book quote: … “with great power comes great responsibility”
ㅤ
WHAT ARE THE LIMITATIONS OF THIS STUDY?
ㅤ
While DeepFace sets a new standard in face verification, it is essential to acknowledge its limitations.
ㅤ
Dependence on Large Datasets:
Limitations: The success of DeepFace heavily relies on the availability of a massive labeled dataset comprising four million facial images.
Impact: This requirement can be a significant barrier for others attempting to replicate or build upon this work, especially those without access to such extensive data.
ㅤ
High Computational Resources:
Limitation: Training the deep neural network (DNN) and performing 3D face alignment requires substantial computational power.
Impact: The resource-intensive nature of the training process may limit its feasibility for smaller organizations or researchers with limited computational resources.
ㅤ
Generalization to Unseen Environments:
Limitation: While the model generalizes well across various datasets, there may still be challenges in adapting it to specific real-world scenarios not covered in the training data.
Impact: The model’s performance might degrade in environments with conditions significantly different from those in the training dataset, potentially limiting its applicability.
ㅤ
Reliance on Precise Instrumentation:
Limitation: The study utilizes advanced techniques like 3D face alignment, which depend on precise instrumentation and accurate initial face detection.
Impact: In real-world applications where such high-fidelity instruments are not available, the performance of the system might be compromised.
ㅤ
Potential Algorithmic Bias:
Limitation: The dataset used for training might not fully represent the diversity of the global population.
Impact: This can lead to algorithmic bias, where the system performs better on certain demographic groups than others, raising ethical and fairness concerns.
ㅤ
Scalability Issues:
Limitation: The model’s complexity and size could pose scalability issues when deploying it in real-time systems or on devices with limited processing power.
Impact: This could hinder the practical deployment of DeepFace in scenarios requiring real-time face verification on edge devices or in low-power environments.
ㅤ
Security and Privacy Concerns:
Limitation: The use of such advanced face recognition systems raises significant security and privacy concerns.
Impact: Without proper safeguards, the technology could be misused, leading to unauthorized surveillance, data breaches, or other privacy violations.
ㅤ
Lack of Addressing Ethical Implications:
Limitation: The paper focuses primarily on technical advancements and does not thoroughly address the ethical implications of deploying such powerful face recognition technology.
Impact: The societal impact, potential for misuse, and ethical considerations remain areas that need more in-depth exploration and discussion.
ㅤ
These limitations highlight the need for further research to address these challenges and ensure that the technology can be safely and effectively integrated into real-world applications.
ㅤ
Transformative Applications
The implications of DeepFace extend far beyond academic achievements. In the realm of security systems, enhanced face verification can significantly improve access control and surveillance, ensuring safer environments. Social media platforms can utilize this technology for more accurate tagging and content management, providing a seamless user experience.
ㅤ
In law enforcement, DeepFace can assist in identifying individuals from images and videos, aiding in forensic investigations and enhancing public safety. Moreover, in healthcare, the technology can be used for patient identification and monitoring, improving the efficiency and accuracy of medical services.
ㅤ
A GLIMPSE INTO THE FUTURE:
ㅤ
The advancements made by DeepFace not only close the gap to human-level performance but also set a new benchmark for what is achievable in face recognition. This work demonstrates the immense potential of coupling 3D modeling with deep learning, offering a blueprint for future innovations in computer vision and artificial intelligence.
ㅤ
As we continue to explore and refine these technologies, the possibilities for real-world applications are boundless. DeepFace exemplifies how far we have come in our quest to create intelligent systems that can see and understand the world as we do, and it hints at an exciting future where AI seamlessly integrates into every aspect of our lives.
ㅤ
CONCLUSION:
ㅤ
The study represents a significant milestone in the field of facial recognition technology. By integrating advanced deep neural networks (DNN) with precise 3D face alignment, the researchers achieved an impressive 97.35% accuracy on the Labeled Faces in the Wild (LFW) dataset, approaching human-level performance. This remarkable feat underscores the potential of combining deep learning with innovative modeling techniques to enhance the reliability and accuracy of face recognition systems.
ㅤ
The study not only demonstrates the technical capabilities of DeepFace but also highlights its transformative applications in various sectors, including security, healthcare, and social media. However, it also brings attention to important ethical and privacy concerns, emphasizing the need for robust safeguards and ethical guidelines to ensure responsible deployment.
ㅤ
While the study’s reliance on large datasets and high computational resources presents certain limitations, the advancements made set a new benchmark for future research. The potential for real-world applications is vast, promising more secure, efficient, and reliable face recognition systems. As we continue to refine these technologies, the lessons learned from DeepFace will guide the development of more advanced AI systems, ultimately contributing to a future where AI seamlessly integrates into our daily lives, enhancing both convenience and security.
Kacper Malinos
ㅤ